Mohamed El Banani
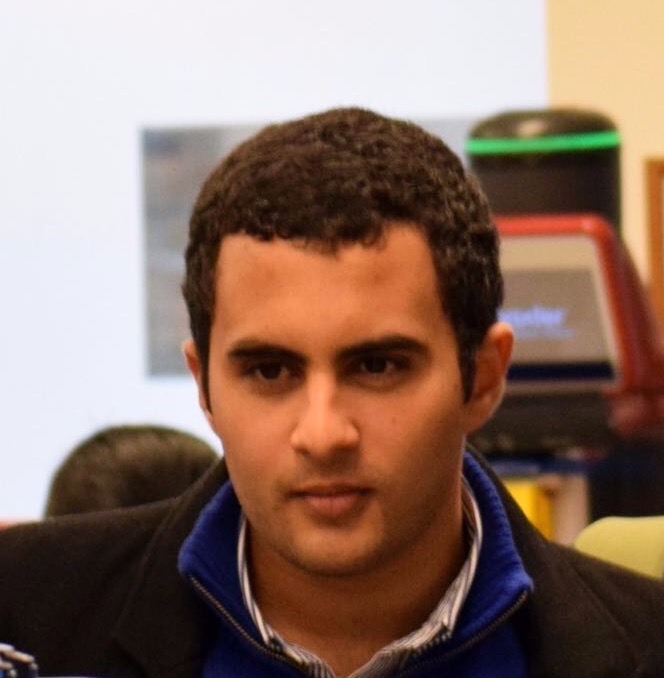
I am a Ph.D. candidate in Computer Science at the University of Michigan working with Justin Johnson.
I am broadly interested in computer vision, machine learning, and cognitive science. My goal is to build systems that learn to represent their visual world without supervision and generalize to novel objects and scenes.
During my PhD, I was fortunate to work with David Fouhey and John Laird at UM, Benjamin Graham at FAIR, and Varun Jampani at Google Research. I did my undergraduate studies at Georgia Tech where I got the chance to work with Maithilee Kunda and Jim Rehg on cognitive modeling, as well as Omer Inan and Todd Sulchek on biomedical devices.
news
Oct 2023 | I gave a talk at the Stanford Vision and Learning Lab on cross-modal correspondence. |
---|---|
Sep 2023 | I chatted in Arabic with Abdelrahman Mohamed about my research. (video on AI بالمصري) |
May 2023 | I am spending the summer at Google Research working with Varun Jampani. |
Apr 2023 | I am serving as a mentor for the Fatima Fellowship. |
Feb 2023 | Our work on language-guided self-supervised learning was accepted at CVPR 2023. |
publications
-
Learning Visual Representations via Language-Guided SamplingIn CVPR, 2023TL;DR: A picture is worth a thousand words, but a caption can describe a thousand images. We use language models to find image pairs with similar captions, and use them for stronger contrastive learning.
-
Self-Supervised Correspondence Estimation via Multiview RegistrationIn WACV, 2023TL;DR: Self-supervised correspondence estimation struggles with wide-baseline images. We use multiview registration and SE(3) transformation synchronization to leverage long-term consistency in RGB-D video
-
UnsupervisedR&R: Unsupervised Point Cloud Registration via Differentiable RenderingIn CVPR, 2021 (Oral)TL;DR: Can we learn point cloud registration from RGB-D video? We propose a register and render approach that learns via minimizing photometric and geometric losses between close-by frames.
-
Novel Object Viewpoint Estimation through Reconstruction AlignmentIn CVPR, 2020TL;DR: Humans can not help but see 3D structure of novel objects, so aligning their viewpoints becomes very easy. We propose a reconstruct-and-align approach for novelobject viewpoint estimation.
-
A Computational Exploration of Problem-Solving Strategies and Gaze Behaviors on the Block Design Task.In CogSci, 2016TL;DR: We present a computational architecture to model problem-solving strategies on the block design task. We generate detailed behavioral predictions and analyze cross-strategy error patterns.
-
A Pilot Study of a Modified Bathroom Scale To Monitor Cardiovascular Hemodynamic in PregnancyJournal of the American College of Cardiology, 2016TL;DR: We use ballistocardiogram measurements extracted from a modified bathroom scale to analyze maternal cardiovascular adaptation during pregnancy for low-cost detection of preeclampsia.